Engineering Efficiency Explained
Featured
"Is our boss SPYING on us?" Developers vs Managers
Can team productivity be tracked without spying on your software developers? Bob and Spike seek to answer this question as they get to the bottom of the measuring manager mystery.
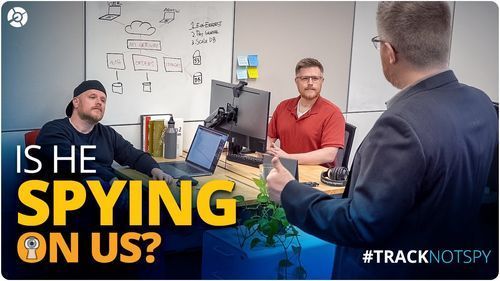